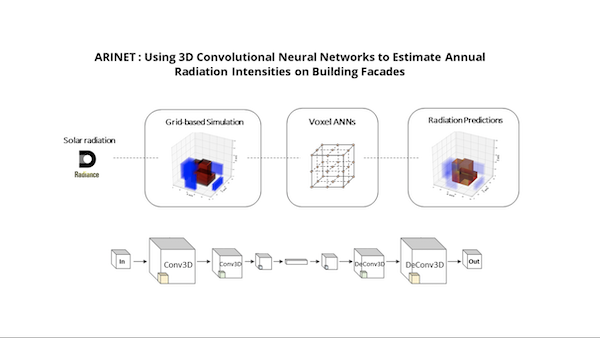
AriNet: Using 3D Convolutional Neural Networks to Estimate Annual Radiation Intensities on Building Facades
Artificial intelligence and data-driven modeling are becoming more prominent in the building and construction sectors. Physics-based models usually require significant computational power and a considerable amount of time to simulate output. Therefore, data-driven models for predicting the physical properties of buildings are becoming increasingly popular. The objective of this research is to introduce Artificial Neural Network (ANN) methods to represent the physical properties of buildings. Achieving this goal will illustrate the future capacity of integrated neural networks in building performance simulations. The Annual Radiation Intensity Neural Network (ARINet) demonstrates the feasibility of using a 3D convolutional neural network to predict the surface radiation received by building façades. The structure of ARINet is composed of 3D convolution, fully connected, and 3D deconvolution layers. This research was trained on 1,635 datasets and validated by 408 datasets generated by a physical simulator. ARINet showed errors in 0.2% of the validation sets.
Learning Objectives:
After attending this presentation, participants will be able to…
Jung Min (Ellie) Han is a doctoral student at Harvard Graduate School of Design and a research assistant at Harvard Green Buildings and Cities. Han seeks strategies for architectural sustainability and energy-efficient building design through her research. She innovates on interoperable building performance simulation (BPS) software for architects to use in nimble building performance analysis and flexible early-stage design decision making. Her doctoral research focused on the geometric properties of architecture and exchangeable data formats for evaluating building designs. Artificial intelligence and deep learning are her primary methodologies for advancing the feasibility of BPS software.
- Interpret radiation simulation results for use in design decision-making.
- Describe the deep learning-based radiation simulation workflow.
- Understand the 3DCNNs architecture and the underlying principles.
- Propose the ANNs-based simulator as an alternative to a physics-based model.
